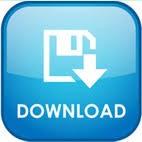
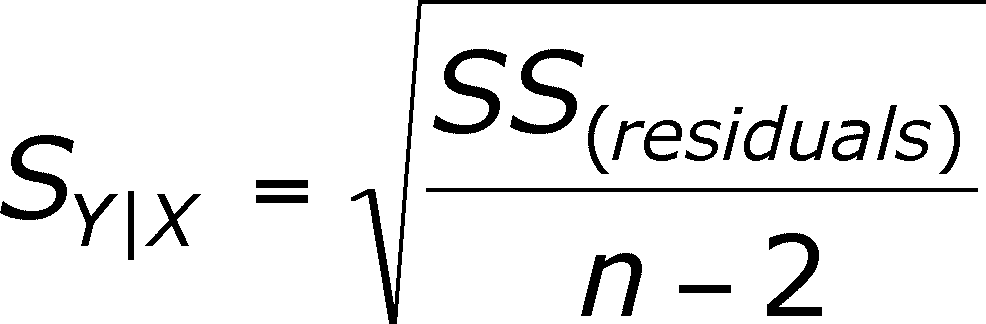
R-Squared can also be represented using the following formula: Pay attention to the diagram and note that the greater the value of SSR, the more is the variance covered by the regression / best fit line out of total variance (SST). Diagrammatic representation for understanding R-Squared Here is a visual representation to understand the concepts of R-Squared in a better manner. As the value of R-squared increases and become close to 1, the value of MSE becomes close to 0. If the value of R-Squared becomes 1 (ideal world scenario), the model fits the data perfectly with a corresponding MSE = 0. Greater the value of R-squared would also mean a smaller value of MSE. For the training dataset, the value of R-squared is bounded between 0 and 1, but it can become negative for the test dataset if the SSE is greater than SST. R-Squared is also termed as the coefficient of determination. This would be discussed in one of the later posts. This is where the adjusted R-squared concept comes into the picture. However, we need to take caution while relying on R-squared to assess the performance of the regression model. The greater the value of R-Squared, the better is the regression model as most of the variation of actual values from the mean value get explained by the regression model. R-squared value is used to measure the goodness of fit or best-fit line. The sum of squares total (SST) represents the total variation of actual values from the mean value of all the values of response variables. Sum of Squares Regression (SSR) represents the total variation of all the predicted values found on the regression line or plane from the mean value of all the values of response variables. R-Squared is the ratio of the sum of squares regression (SSR) and the sum of squares total (SST). Mean Squared Error Representation What is R-Squared? Here is the diagrammatic representation of MSE for a simple linear or univariate regression model: Fig 2. In the above equation, Y represents the actual value and the Y_hat represents the predicted value that could be found on the regression line or plane. RMSE has also been termed root mean square deviation ( RMSD). When you take a square root of MSE value, it becomes root mean squared error (RMSE).
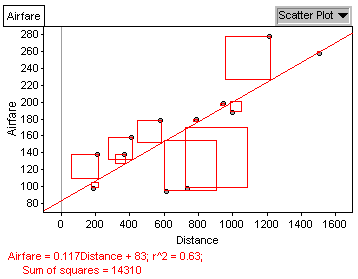
A value close to zero will represent better quality of the estimator/predictor (regression model).Īn MSE of zero (0) represents the fact that the predictor is a perfect predictor.

This is how it is represented mathematically: Fig 1. It is also termed as mean squared deviation (MSD). Mathematically, the MSE can be calculated as the average sum of the squared difference between the actual value and the predicted or estimated value represented by the regression model (line or plane). And, the functions that are not smooth are difficult to work with when trying to find closed-form solutions to the optimization problems by employing linear algebra concepts. The absolute value of error is not convenient, because it doesn’t have a continuous derivative, which does not make the function smooth. The question that may be asked is why not calculate the error as the absolute value of loss (difference between y and y_hat in the following formula) and sum up all the errors to find the total loss. In 1805, the French mathematician Adrien-Marie Legendre, who first published the sum of squares method for gauging the quality of the model stated that squaring the error before summing all of the errors to find the total loss is convenient. The Python or R packages select the best-fit model as the model with the lowest MSE or lowest RMSE when training the linear regression models. When the linear regression model is trained using a given set of observations, the model with the least mean sum of squares error (MSE) is selected as the best model. The lesser the MSE, the better the regression model is. Two or more regression models created using a given sample of data can be compared based on their MSE. And, the squared loss (difference between true & predicted value) is advantageous because they exaggerate the difference between the true value and the predicted value. In other words, it can be used to represent the cost associated with the predictions or the loss incurred in the predictions. Intuitively, the MSE is used to measure the quality of the model based on the predictions made on the entire training dataset vis-a-vis the true label/output value.

The Mean squared error (MSE) represents the error of the estimator or predictive model created based on the given set of observations in the sample. Difference between Mean Square Error & R-Squared.
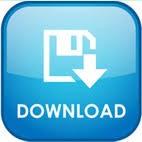